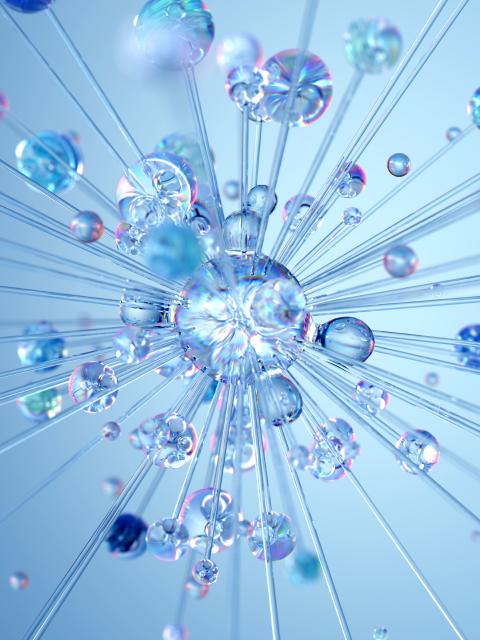
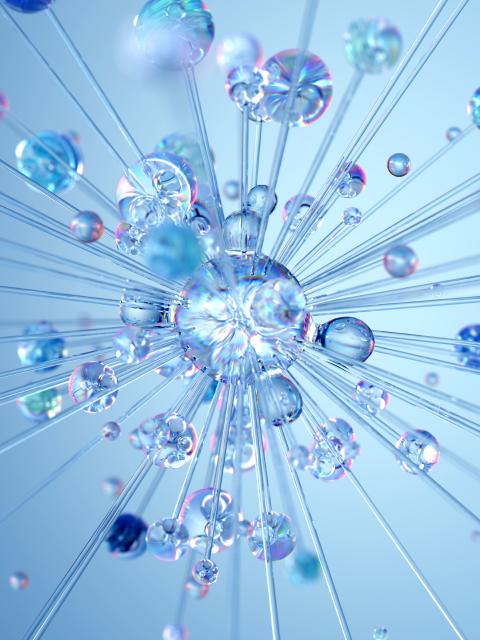
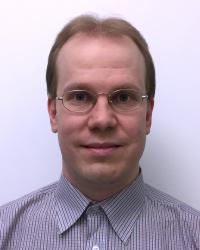
Lead Data Scientist
Mobile network KPIs are collected from the radio access network, and they provide continuous information on the performance of the system. At the cell level, performance management data can be used to identify degraded quality of the service caused by diverse factors, such as faulty equipment, interference from nearby cells, inadequate updates in the configuration parameters, and more.
Monitoring and correcting network performance using AI and automation is a smart move from a straight-up business perspective. Monitoring and detecting when adverse changes occur in the network KPIs is critical for optimal operation and maintaining adequate service for users. When the quality of mobile service improves, it naturally increases customer satisfaction as well as reducing the time spent identifying the root causes of performance degradation.
Furthermore, in an ever-expanding, complex and dynamic network, the number of KPIs is so high that monitoring them manually and taking corrective action is not even really possible. In order to remain competitive in a landscape of increasing complexity, it is necessary to adopt increased automation through intelligent algorithms. This facilitates more autonomous and cost-effective operations overall.
Dynamic networks have dynamic problems
Operators have identified some thresholds in network KPIs beyond which customer satisfaction becomes significantly affected. For example, throughput rates below 5 Mbit/s tend to result in increased dissatisfaction among users, leading to degraded customer and brand experience and, at worst, customer changing operator.
The demands placed on a network constantly vary as devices join and leave, and as devices move between cells. On top of this, equipment is changed and updated, and of course, there are inevitable faults and breakdowns.
These kinds of changes in the mobile network – planned and unplanned – result in an increased need for operators to monitor network behaviour and performance. With 5G and the Internet of Things, the number of devices accessing mobile networks is also growing rapidly, increasing the load on networks. That also adds to the difficulty of monitoring performance, increasing the risk of degraded performance that once again impacts customer satisfaction.
Network operation centres (NOCs) receive alarms directly from network equipment when something is not working as it should be. Alarms are normally defined by the equipment manufacturer, and they are a useful tool for dealing with hardware and software faults.
A standard, personnel-operated NOC can only respond to alarms that are triggered by software or hardware. However, due to the dynamic nature of networks, there can be many reasons for degraded performance that aren’t related to software or hardware glitches and thus go undetected by NOC staff.
Performance alarms provide next-level solutions
There are many challenges in manual KPI monitoring, ranging from insufficient data on performance changes in the network and the reactive nature of troubleshooting, to slow root cause analysis and fixed threshold monitoring, which is unsuitable for monitoring every cell in the network.
As there are over 100,000 cells in Elisa’s mobile network, monitoring personnel are tasked with monitoring dozens of KPIs – such as success rate, drop rate, packet loss, and throughput – in various cells simultaneously, in the worst case. Continuously keeping track of potentially millions of data sets and spotting anomalies is not only impractical and financially non-viable, but in the end, basically impossible when the desired result is first-rate network performance.
In reaching that level of performance, one crucial metric is the quality of the anomalies found in the network. Using AI and machine learning, we are able to switch to adaptive monitoring with data on unique cell characteristics, instead of using fixed thresholds. This provides a more flexible and granular way of monitoring performance based on detecting the point at which something changes and begins to degrade, leading to finding the pertinent anomalies and fixing them to increase performance and service quality.
Key to all of this are performance alarms generated by AI from previously detected anomalies, and not necessarily based on software or hardware malfunctions. Performance alarms can trigger, for example, automatic resets of network equipment based on KPI data or, when that does not solve the problem, notify a human operator through an interface. In the future, it will also be possible to perform automated root cause analysis to generate automated fixes for network equipment.
When an ML algorithm is trained to spot anomalies using historical time series data, it is able to monitor very gradual changes in trends, or sudden and sustained step changes that could otherwise easily go unnoticed. This kind of statistical change point detection is one of the main goals of automated mobile network KPI troubleshooting.
There is also the potential for using ML algorithms to make forecasts, perhaps even predicting issues before they start to occur.
Getting to focus on what is essential
Now that we have a virtual NOC with AI-powered anomaly detection and performance alarms, we begin to get radically increased visibility over network KPIs, the possibility to find and resolve problems proactively, faster root cause analysis, and adaptive thresholds based on unique cell characteristics. Once the ML algorithm is properly configured and trained and its parameters have been tuned, it can continuously monitor a selected set of relevant KPIs network-wide based on statistical modelling.
The concrete benefits show as early warnings of degraded performance that can be immediately fixed. In a modern, dynamic network environment, the ability to detect issues that are not directly related to hardware or software glitches and to identify root causes quickly is a clear advantage. That obviously also translates to a better customer experience.
This also means that we can detect anomalous performance faster and potentially react in a very tight timeframe, rather than waiting for a threshold to be passed or for a customer to submit a complaint.
AI and ML assist human experts by helping them identify the issues that are the most relevant and prioritising them, at the same time allowing them to focus on what is essential, rather than routine monitoring
This kind of solution permits Elisa to reduce the time it takes to respond to anomalies in the network and to enhance customer experience and brand experience, resulting in fewer customers leaving and even more new, satisfied customers.